- Scientists have deployed an artificial intelligence model to identify and detect roads in rural and remote areas.
- The model was trained to analyze satellite images and pick out the roads within them; according to a recent study, it managed to do this accurately eight times out of 10.
- Road construction has increased drastically in recent decades, with 25 million kilometers (15.5 million miles) of paved roads expected to be built by 2050.
- Illegal roads, which fall outside the purview of environmental governance, often cut through dense forests and cause harm to the biodiversity living in fragile ecosystems.
For years, detecting illegal roads in remote areas has remained a challenging and labor-intensive task. More often than not, it requires poring over satellite images to identify thin lines cut through the dense green of forests and fragile ecosystems.
Enter artificial intelligence.
A new study published in the journal Remote Sensing describes how scientists have automated the process of mapping illegal roads that are wreaking havoc on the environment. The model was trained to detect roads from satellite images captured from rural and semiforested areas in Papua New Guinea, Malaysia and Indonesia. According to the study, the model identified roads with an accuracy of up to 81%.
“The forests in those areas are very dense, and oftentimes, there might be a logging road or some illegal road that you wouldn’t be able to clearly see,” study co-author Bill Laurance, a distinguished research professor at James Cook University in Australia, told Mongabay in a video interview. “But artificial intelligence and computer models can be trained to do that.”

There’s been an unprecedented increase in road building in the past few decades. Twenty-five million kilometers (15.5 million miles) of paved roads are expected to be built by 2050, with previous research showing that nine-tenths of all road construction is happening in countries in the Global South.
“We are living in the most dramatic era of road expansion in human history,” Laurance said. “Many of these roads are degrading ecosystems because they’re opening them up like a flayed fish to illegal activities and exploitation.”
Illegal roads, in particular, pose an increased threat because they don’t fall within the purview of environmental governance. The gaps that exist in detecting these roads have led to a blind spot in the data required for conservation and advocacy efforts. Citing previous research, Laurance said road-mapping studies in the Brazilian Amazon and Asia Pacific have found up to 13 times more road length than reported in government databases.
“They are proliferating much faster than we can possibly map them using the human eye,” he said.
As part of their research, Laurance and his team worked with volunteers to manually map roads from high-resolution satellite images. They then fed the same data set, consisting of approximately 8,900 images, into their artificial intelligence model to train, validate and test it. Their model is based on convolutional neural networks, an AI methodology widely used for image analysis and identification.
“The training process involved visual interpretation of these images,” co-author Tao Huang, a senior lecturer in electronics systems and IoT [internet of things] engineering at James Cook University, told Mongabay by email. “It takes only a few hours to train the model as we divided the larger imagery into smaller ones.”
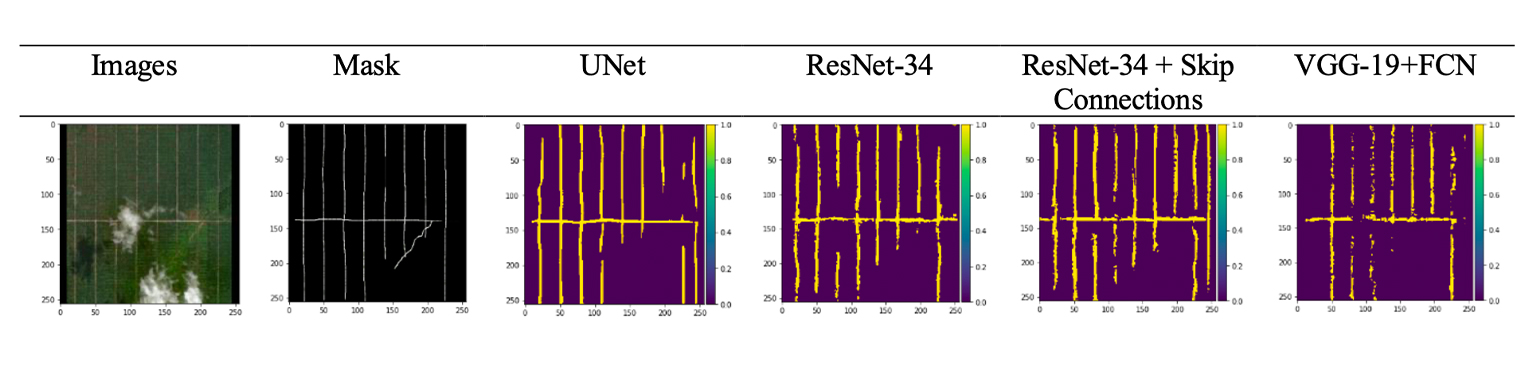
They fed the model satellite images, and it attempted to detect any roads within them. It then produced maps that showed the roads it had identified, agreeing with the manually identified roads eight times out of 10. The team is currently working on a related study that will include more data on the geographical locations and distribution of the roads detected over the course of the research.
Huang said training an AI model to detect irregular, rustic and often obscure roads in remote areas was not an easy task. For anyone attempting something similar in the future, he emphasized the importance of high-resolution imagery.
“My advice would be to focus on acquiring high-quality and diverse datasets for training, and consider the unique characteristics of the area of interest,” he said. “And use a robust evaluation framework to assess the performance of the model.”
Banner image: Illegal road leading to an oil palm plantation in Indonesia. Image by Rhett A. Butler/Mongabay.
Abhishyant Kidangoor is a staff writer at Mongabay. Find him on 𝕏 @AbhishyantPK.
New funding boosts AI-enabled wildlife identification project in Australia
Citation:
Sloan, S., Talkhani, R. R., Huang, T., Engert, J., & Laurance, W. F. (2024). Mapping remote roads using artificial intelligence and satellite imagery. Remote Sensing, 16(5), 839. doi:10.3390/rs16050839