- An artificial intelligence project to identify native species from camera-trap images in Australia has received a A$750,000 ($492,000) grant from the government.
- The project by the conservation nonprofit Australian Wildlife Conservancy has already trained the AI model to identify 44 species from camera-trap images, from native animals such as kangaroos and dingoes, and invasive ones like cats and foxes.
- With the new funding, the team at AWC aims to scale up the model to be able to identify 120 species; the model can also be applied to alert land managers about invasive predators that are harmful to native species.
- Camera traps continue to be one of the most widely used technologies for biodiversity surveys, but the analysis of the thousands of images generated by camera traps remains a major bottleneck.
In 2019, the conservation nonprofit Australian Wildlife Conservancy started developing an artificial intelligence model to identify animals in camera-trap images. Since then, despite limited funding and resources, the team has managed to train the model to recognize 44 species, from native dingoes, kangaroos and woylies, to introduced predator cats and foxes.
Now, thanks to a funding grant from the Australian government, the project is getting a boost that will enable it to almost triple the model’s capabilities. The A$750,000 ($492,000) grant from the government’s Innovative Biodiversity Monitoring Grants Program will help AWC further develop the model to recognize and identify up to 120 native species.
“The funding will allow us to employ additional staff to gather the source data, train and test the models, and purchase additional AI-processing power in the cloud,” Damien Kerr, chief information and technology officer at AWC, told Mongabay in an email interview.
Camera traps have long been used for wildlife tracking and monitoring. Even as newer and more cutting-edge technology have been developed and deployed globally, camera traps continue to be one of the most widely used tools for biodiversity surveys. However, as their capabilities have increased over the years, so has the amount of data they gather in a short span of time. For scientists and researchers, analyzing and processing these images to identify individual species remains a major bottleneck. Not only is the process laborious and time-consuming, but it also requires expertise to recognize the animals accurately.
“While species like koalas and echidnas are easily recognizable, it can take a lot of practice to confidently distinguish a bush rat from a swamp rat,” Holly Sitters, one of the leads in the AWC project and senior wildlife ecologist, told Mongabay in an email interview.
To overcome this shortcoming, the team at AWC decided to incorporate artificial intelligence into their work. “By automating the process of tagging images, it will be possible to generate results in a fraction of the time it takes when processing images manually,” Sitters said. “This means that information can be fed more quickly to land managers.”
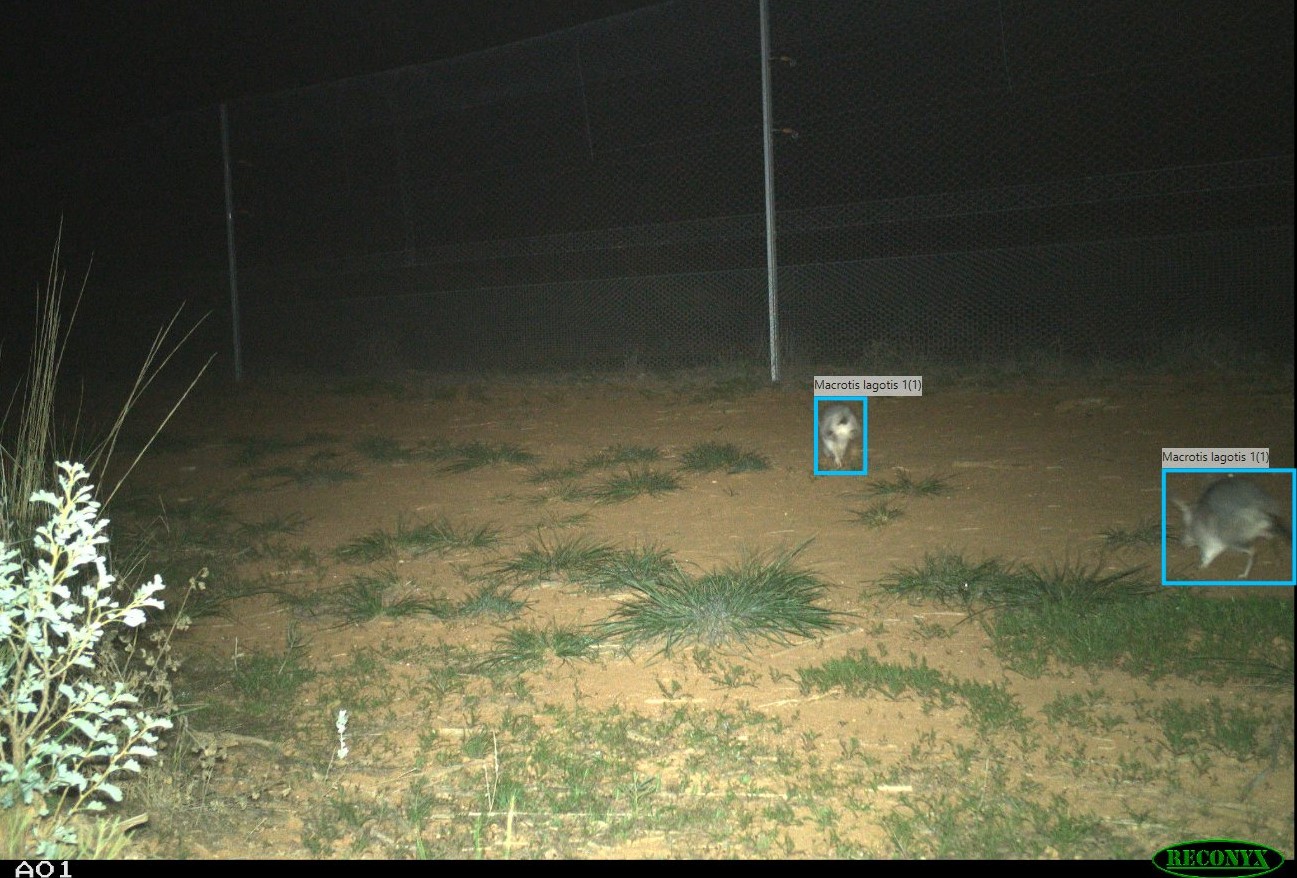
To train the model, the team at AWC has been using data from the organization’s library of camera-trap images collected over the years from their network of sanctuaries. For each species, they use close to 1,000 high-quality images to train the model. “The training images must show the species from a range of angles, under a range of light levels, and ideally in a range of habitats,” Sitters said. Once the model is trained, “species classifications from our national network of experts will be compared with the AI classifications to measure its success, and refinements to the models will be made where necessary,” she said.
While the model can be used to monitor and study native species, it also has an application in helping identify harmful invasive species. “For example, the presence of an introduced animal at a site may trigger prompt management action to prevent harm to native species,” Sitters said.
With the federal funding, the team plans to bring in new staff and resources to scale up the number of species that can be identified by the model. While obtaining thousands of images of each species, especially rare and threatened ones, will be a challenge, Kerr said the funding will help the team establish guidelines and standards to maintain consistency across a range of sources and testing metrics.
“While species recognizers of very common fauna are commonplace, models of rare, threatened or endangered species are much harder to build with high performance, and it is precisely those species that are in need of monitoring,” Kerr said. “We aim to release models that contain such species for conservation, and ultimately to allow future technologists and conservationists to build upon.”
Banner image: A Boodie (Bettongia lesueur) and a Bilby (Macrotis lagotis), two Australian marsupials. Image by Philip Bouchard via Flickr (CC BY-NC-ND 2.0).
Abhishyant Kidangoor is a staff writer at Mongabay. Find him on 𝕏 @AbhishyantPK.